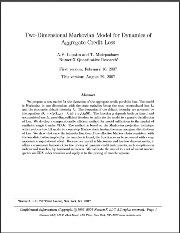
Two-Dimensional Markovian Model for Dynamics of Aggregate Credit Loss
In this article, we propose a new model for the dynamics of the aggregate credit portfolio loss. The model is Markovian in two dimensions with the state variables being the total accumulated loss Lt and the stochastic default intensity λt. The dynamics of the default intensity are governed by the equation dλt=κ(ρ(Lt, t)−λt)dt+σ√λtdWt. The function ρ depends both on time t and accumulated loss Lt, providing sufficient freedom to calibrate the model to a generic distribution of loss. We develop a computationally efficient method for model calibration to the market of synthetic single tranche CDOs. The method is based on the Markovian projection technique which reduces the full model to a one-step Markov chain having the same marginal distributions of loss.
We show that once the intensity function of the effective Markov chain consistent with the loss distribution implied by the tranches is found, the function ρ can be recovered with a very moderate computational effort. Because our model is Markovian and has low dimensionality, it offers a convenient framework for the pricing of dynamic credit instruments, such as options on indices and tranches, by backward induction. We calibrate the model to a set of recent market quotes on CDX index tranches and apply it to the pricing of tranche options.
Authors: Andrei V. Lopatin, and Timur Misirpashaev
In this article, we propose a new model for the dynamics of the aggregate credit portfolio loss. The model is Markovian in two dimensions with the state variables being the total accumulated loss Lt and the stochastic default intensity λt. The dynamics of the default intensity are governed by the equation dλt=κ(ρ(Lt, t)−λt)dt+σ√λtdWt. The function ρ depends both on time t and accumulated loss Lt, providing sufficient freedom to calibrate the model to a generic distribution of loss. We develop a computationally efficient method for model calibration to the market of synthetic single tranche CDOs. The method is based on the Markovian projection technique which reduces the full model to a one-step Markov chain having the same marginal distributions of loss.
We show that once the intensity function of the effective Markov chain consistent with the loss distribution implied by the tranches is found, the function ρ can be recovered with a very moderate computational effort. Because our model is Markovian and has low dimensionality, it offers a convenient framework for the pricing of dynamic credit instruments, such as options on indices and tranches, by backward induction. We calibrate the model to a set of recent market quotes on CDX index tranches and apply it to the pricing of tranche options.
Authors: Andrei V. Lopatin, and Timur Misirpashaev